Is Metrifying Products The Way Moving Forward?
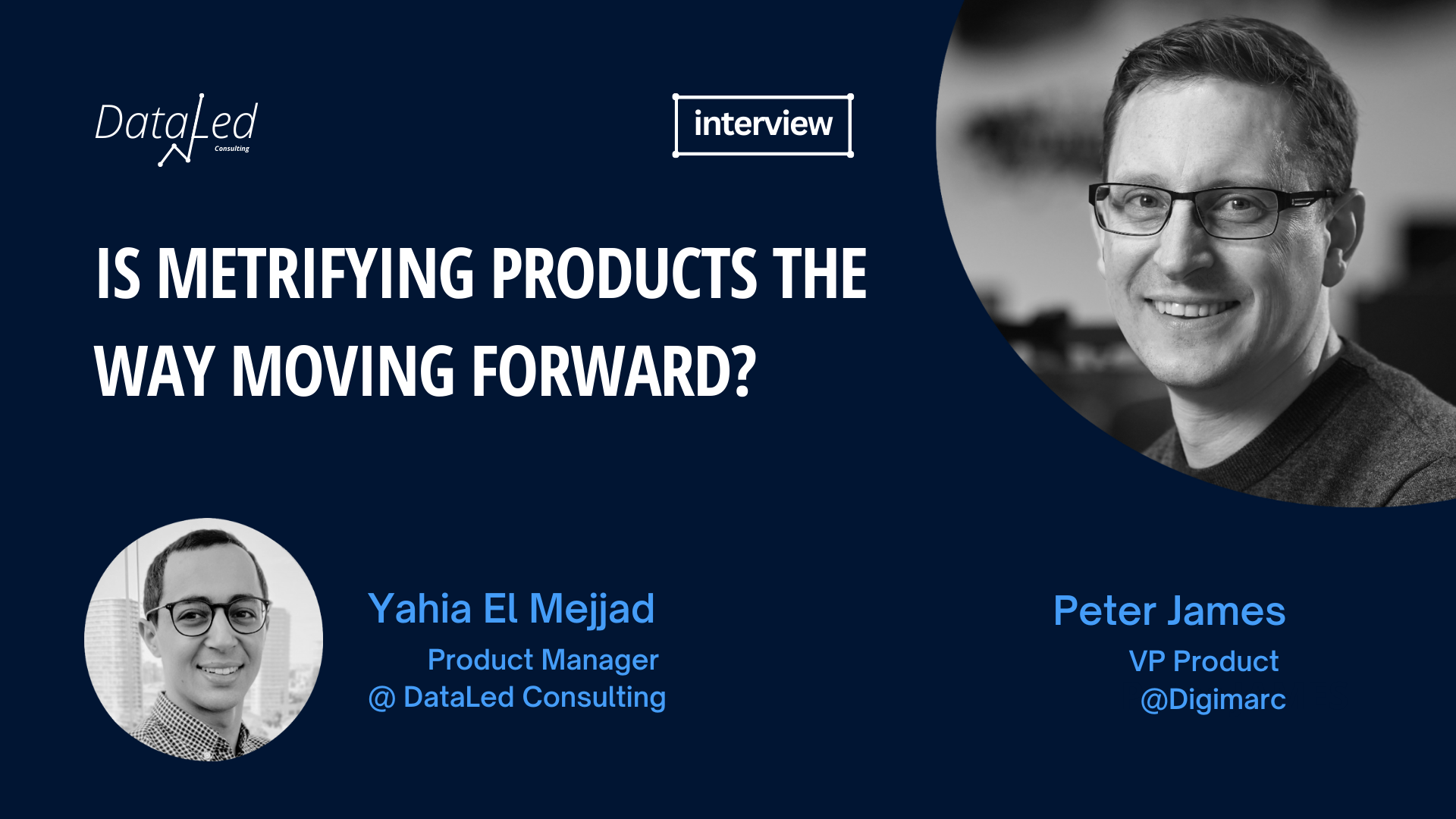
IT companies collect a great amount of data about their products and customer behaviours but often underestimate the power data can have in making better and more informed product decisions.
When it comes to data, there are some important questions to consider. What data is relevant to us and what other data is just noise? What skills do we need to be able to analyse and make sense of this data? What tools should we invest in to be able to visualise good insight? And what about performance?
I invited Peter James, Vice President of Product Strategy at Digimarc to discuss the obstacles a typical product team can encounter while trying to utilise data to make better decisions.
Yahia: What place should data occupy in the process of shaping good products?
In other words, where to put the cursor between no data-based or metric-based decisions on one hand (i.e. customer feedback, discovery sessions) AND fully evidenced decision-making on the other hand?
I like to give an example of a “reset password” feature: Our product is a mobile app, and we think we need to implement a reset password feature. How do we go about evidencing this, or how do we justify that this feature is going to benefit the Customer?
Peter: First of all we need to define what we mean by ‘data’. In your example, I would say that customer feedback and discovery sessions are entirely about data collection, but I think you are referring to product metrics as a subset of ‘data’.
We should be driving all of our decisions from data and that should be part of the full development lifecycle: why are we focusing on certain areas/problems; what priority do we assign to each of these; what is the right solution to the problems we want to address; and once we have a solution in place, is it successful? Sometimes we can only identify areas to focus on from things like customer feedback and discovery sessions because we won’t have infinitely instrumented our systems, or the problem is a missing feature (like your reset password for example) that we will only discover because customers are telling us that they need it. We might also discover this because of a huge number of calls to our service centres asking for passwords to be reset - or worse, seeing customers opening multiple accounts because once they forget their password their only choice is to open another account.
This raises an interesting point around data interpretation and ensuring that you understand the motivations behind customer behaviours before jumping to conclusions about what the issue is. Customer drop off as above could be interpreted as customers losing interest in our product and leaving rather than simply losing access. Product metrics won’t be able to give you information about the motivations. They just observe the behaviour - and so that is where activities like feedback sessions and discovery sessions come into their own and are a required companion to the product metrics.
Yahia: You raise some very interesting points. So, what you are saying is that:
- Data is fundamental to decision-making
- Any piece of information we collect about our customers (be it tracked product metrics, customer feedback, or discovery sessions outcome) is data useful to drive decisions.
- Product metrics alone can be tricky to interpret sometimes without customer feedback and discovery sessions to discuss what happened. You gave a good example of a scenario where there is a customer sign-in drop-off due to forgotten passwords (say), and while tracked metrics will capture a drop-off in the number of sign-ins, the reason behind it can only be understood by speaking to customers directly, otherwise, we can jump to wrong conclusions, and this, again, is data-based decision making.
We both agree that there must be some sort of strong evidenced process before carrying out any implementation work. I appreciate we need to be agile and allow ourselves to implement features and unpack them if necessary, but this process shouldn’t be solely based on intuition. We need to be able to bring evidence to the table as to why the customer is going to benefit from feature X to achieve Y. The biggest piece of evidence can be retrieved from the data: Will feature X offer the customer a better, quicker service and deliver more?
We both agree data is fundamental to product decision-making, but in reality, we know that businesses struggle with it. What’s the challenge or the obstacle getting in our way?
Peter: I don’t think anyone would disagree with the need for making data-based decisions, but unless you have the right tooling, and ease of access to the data to be able to create the insights needed you are going to struggle. And depending on your budgets, pressures, etc. making the changes needed may feel like an impossible task.
I think the challenge is that adding tooling and instrumenting your product might take effort away from developing new features and is so much harder to add retroactively. Teams are usually under pressure to drive revenue or deliver on a roadmap of features that promises to increase revenue. Therefore, it is a difficult pill to swallow to have to postpone that whilst you build your data architecture. Of course, we know that in the long term, it is absolutely the right thing to do and will pay itself back, but we are often caught in a cycle of short-term returns that needs to be satisfied.
There is also the implicit admission that you have been working without data to that point. This could cause embarrassment. No one - especially those in senior or leadership positions - wants to admit that they have been flying blind, although they probably have been using some form of metrics to report success to that point. So why now do they need to suddenly invest time, resources, and money to replace the existing process?
In my experience, a current lack of metrics is the reason behind looking for a new data architecture that tries to cover all bases and be future proofed. I’m not going to say that it is necessarily over engineered but it is likely more than MVP and unlikely to be something that can be developed incrementally - hence the challenges. It is very hard not to be like a kid in a candy shop and want to have all the data that you can get your hands on when you have been starved of it to date. But the reality is that a lean, incremental approach is generally going to be more palatable to the business and deliver value more immediately, allowing you to justify further investment and effort.
Yahia: That’s interesting, so there are various obstacles: tooling, access to data, and other company-related issues such as budget and the approach to data initiatives.
With regards to tooling and access to data, I will also add that, up until recently, our ability to mine data and cross-join tables was limited. Limited by the computing power required to run very complex queries, limited by the tools available to write queries and visualise dashboards, but also by the cost of running these queries. Whereas now, we have a profusion of tools and languages making mining and visualising data a relatively easy task. In fact, businesses started mining data and getting some interesting insights. There are also AI tools now that can classify your data, interpret it and apply machine learning models to predict customer churn for instance.
On the ground though, it’s another story. Product teams don’t have the investment required to acquire these tools and capabilities. And as you mentioned, a team dedicated to implementing features cannot possibly shift its focus to work on data infrastructure and data metrics without impacting its short-term delivery or commitments. Hence, the need for companies to recognise the long-term benefits of investing in Data teams and build a strategy around it.
And this gets me to the approach to tackling data initiatives. You touched on the fact that the lack of metrics leads to ambitious plans to have future-proofed data architectures with dashboards tracking all possible metrics from day 1, whereas an incremental approach is more suitable to achieve these plans. Could you please expand on this? How do you think product teams can overcome the challenges we’ve mentioned, or more specifically, where do you think teams should start?
Peter: My advice is don’t go from nothing to everything in one step - it will likely require too much impact on delivery expectations and - as with all development programs - you simply won’t know what you really need to know to plan the end point accurately. Take a lean approach and identify opportunities for capturing data and measuring success metrics for new features and key workflows/funnels first. Then start to grow your instrumentation from there.
Decide what genuine performance metrics are for your product - don’t be tricked into vanity metrics that don’t add real insight and waste implementation effort. And don’t try to over instrument: measure what matters and stop there. The aim is not to capture all the data, but to use data to create actionable insight. Sometimes too much data can be as paralysing as no data at all because you can’t see the insights amongst all the data.
Get into the habit of developing data insights and learning to recognize your own data. We are almost always looking at how metrics are changing as a result of some stimulus and so must understand our baseline/expected data to be able to interpret what we are seeing. What is ‘normal’ for you? What is meaningful for you? What are current trends in your data? What is the outcome of the latest release and the impact on your ‘norms’?
Platform data is particularly powerful for understanding the impacts and outcomes of work or external stimuli. The measurement of the outcome and the interpretation of that data is as important as the initial insight. Become an expert in your own data first - learn your normal - then you’ll be in a position to interpret changes going forwards.
Yahia: So start small, increment, become an expert of your data and measure outcomes of new features is the way moving forward. I couldn’t agree more. I’d like to elaborate on this to highlight the questions product teams should answer as part of their data initiatives:
- What metrics do we need to track? The answer to this one sounds obvious, but unless you figure out a way to metrify the product (i.e. few metrics should be able to tell you the performance of your Product), you will find yourself tracking unnecessary or irrelevant metrics (the vanity metrics for example). The number of users metric is a good example of vanity metric: While the number of users could indicate how your product is growing, this doesn’t tell you how your product is performing, and whether your customer is happy with it or is getting the full value out of it.
- The skills and tools required to visualise insight: a very common mistake is to invest in BI tools alone and give access to everyone in the team thinking that this will be enough to build insight. BI tools are important and all claim they are easy to use (as in drag and drop) to build a dashboard, but in reality, these tools lack the insight and intelligence that is hidden in your DB tables.This means that you will still need some tech expertise in writing complex queries and organising data that goes hand in hand with your BI skills.
- How to visualise data? I wrote an article (9 Rules For An Actionable Product Dashboard) on the subject. I think most companies spend more time making sure their dashboards look beautiful and well-designed than insightful. I made the point that there are 7 (but effectively only 3) types of visuals you need to pick up from when visualising. I also talked about the audience: your visual is intended for a specific audience and not everyone.
- Performance: I believe that Product teams sometimes get overzealous when it comes to dashboards performances. Your Product Performance dashboard is intended to be used internally to bring about some very interesting insight. Often, the reason why these dashboards are slow is that they perform very complex queries made of joins that go through big sets of data. While their performance should be adequate, it doesn’t need to be down to the millisecond. Your dashboard needs to be refreshed at most daily. A daily refresh that takes minutes or even a couple of hours to run is enough to make good product decisions.
- Educating teams: In my view the most difficult one. We need to educate teams to trust their data better than their intuition and to use your own words: you need to become an expert of your data. Today, we can get interesting insight from our data, tomorrow we will be able to get intelligence. So we need to be prepared.